I put OpenAI's new o3-mini model to the test — and the results are staggering
OpenAI's newest model has some issues
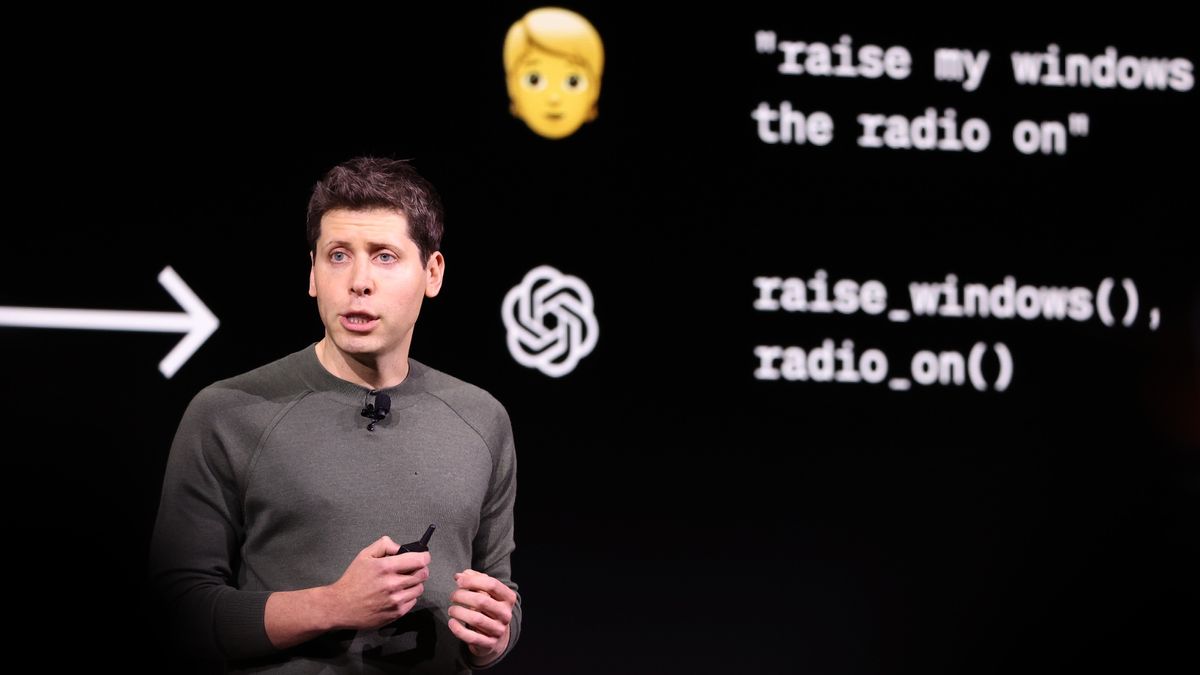
OpenAI has just released o3-mini, a new reasoning model which offers the same kind of performance as its earlier o1 model, but at a fraction of the cost. The new product has garnered praise for its efficiency and speed, and has launched near the top of the benchmark tables across the world.
Reasoning models are all the rage at the moment, and justifiably so. These AI products take their time to evaluate their responses, rather than spitting out the first answer they come up with.
It means a slightly longer wait for an answer, but hopefully a more accurate response with zero hallucinations.
So is all the hype about reasoning valid, and if so does o3 add to the genre, or is it more of the same old stuff wrapped up in a shiny new ribbon?
There are three reasoning levels (or effort) you can set for the model — low, medium and high.
I decided to do a couple of tests of o3-mini to try and get a feel for the quality, value and utility of the new release. As usual I’ve stayed away from benchmarks, because in my opinion they only tell a part of the story with regard to model quality.
There are three reasoning levels (or effort) you can set for the model — low, medium and high. I decided to just test high and low to get an idea of performance at both ends of the spectrum.
Test 1: Truth or Lie?
The prompt: A TV game show contestant stands in front two boxes. Box 1 contains the keys to the star prize of a new car, Box 2 holds an apple. There are two game show hosts, — one always tells the truth, and one always lies — but she doesn’t know which is which. She is only allowed to ask one question to one of the hosts to find out which box holds the prize.
Sign up to get the BEST of Tom's Guide direct to your inbox.
Get instant access to breaking news, the hottest reviews, great deals and helpful tips.
Question: What single question should she ask, and how can she use the response to choose the right box?
Answer: The answer should be for her to ask one host — "If I asked the other host which box has the keys, what would they say?”
Verdict
The o3 model nailed the answer extremely easily, using both high and low reasoning. On high reasoning it took 5424 milliseconds, using 867 tokens for the answer. On low, it took 3157 ms, and 231 tokens output. Quite a difference in effort.
The reasoning is, of course, that regardless of who she asks, the answer given will always indicate the wrong box. So she has to choose the opposite box to whatever she’s told.
Test 2: Race Fuel
I have to give credit to this Reddit thread and Hacker News for this one.
The prompt: I'm playing the Assetto Corsa Competizione racing game. My qualifying time was 2:04.317, the race is 20 minutes long, and the car uses 2.73 liters per lap.
Question: I need you to tell me how many liters of fuel to take for a race.
Answer: You need 27.3 liters, bonus for adding a little extra for safety.
Verdict
To be correct, the reasoning involves calculating all the race times in seconds, estimating the number of laps (total race time divided by lap time), and deriving the fuel from that. You cannot do a partial lap, of course.
This time the o3 model on low reasoning — it’s weakest setting — got the correct answer in 5647 ms and 328 tokens. Shockingly, it got the answer wrong on its most powerful high reasoning setting.
Even worse, it took a whopping 10.9 seconds and 1918 output tokens to get an incorrect answer. o3-mini on high said 26.3 liters rounded up to ‘about 27’.
To put this into perspective, DeepSeek R1 got the correct answer first time in 29 seconds. Even the tiny Qwen2.5:7B model, which I ran locally on my home computer, got a credibly close answer in 15.8 seconds. Qwen 2.5 7B said 27.03 liters or ‘approximately 27 – 28 liters’.
To say I’m staggered is an understatement.
Bottom line
This is far from from a scientific test, but it’s a fascinating example of just how careful we all should be when relying on supposed ‘state of the art’ AI models for our decisions.
Yes, it's a silly little example, but it just shows what the real issue is with the current state of AI development. The hype boldly talks about AGI and ASI, and yet it’s clear we still can’t be 100% confident on even the most basic questions.
It’s yet another example of the how many r’s in strawberry debacle, which many LLMs originally got wrong. One has to wonder just how long it’s going to be before an AI causes a serious incident somewhere around globe, which is neither trivial or inconsequential. Time will tell.
More from Tom's Guide
- I just tested ChatGPT's new o3-mini model with 7 prompts to rate its problem-solving and reasoning capabilities — and it blew me away
- OpenAI introduces new features for WhatsApp users — here’s what’s new
- 5 tips for getting the most out of MidJourney











Nigel Powell is an author, columnist, and consultant with over 30 years of experience in the technology industry. He produced the weekly Don't Panic technology column in the Sunday Times newspaper for 16 years and is the author of the Sunday Times book of Computer Answers, published by Harper Collins. He has been a technology pundit on Sky Television's Global Village program and a regular contributor to BBC Radio Five's Men's Hour.
He has an Honours degree in law (LLB) and a Master's Degree in Business Administration (MBA), and his work has made him an expert in all things software, AI, security, privacy, mobile, and other tech innovations. Nigel currently lives in West London and enjoys spending time meditating and listening to music.